Site menu:
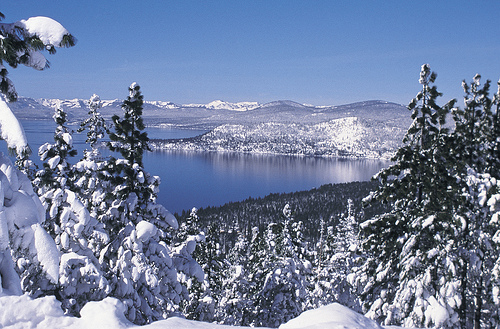
Optimization@NIPS
We welcome you to participate in the 6th NIPS Workshop on Optimization for Machine Learning, to be held at:
Lake Tahoe, Nevada, USA, Dec 9th, 2013
Call for Participation
We invite participation in the 6th International Workshop on "Optimization for Machine Learning", to be held as a part of the NIPS 2013 conference.
Research contributions from the community form an integral part of our program and we invite papers for oral and poster presentation in the workshop. We encourage authors to not only submit finished pieces of work, but also works currently in progress that you would like to announce and get feedback on.
To encourage authors to submit cutting-edge work, the workshop will offer a best paper award as recognition. We also encourage submissions describing practical systems and softwares that have been implemented to address various optimization problems that arise in machine learning.
Note: OPT2013 does not prohibit dual submission; as long as your original venue is ok with it, feel free to submit to OPT2013.
The main topics are, including, but not limited to:
- Stochastic, Parallel and Online Optimization:
- Large-scale learning, massive data sets
- Distributed algorithms
- Optimization on massively parallel architectures
- Optimization using GPUs, Streaming algorithms
- Decomposition for large-scale, message-passing and online learning
- Stochastic approximation
- Randomized algorithms
- Nonconvex Optimization
- Nonsmooth, nonconvex optimization
- Nonconvex quadratic programming, including binary QPs
- Convex Concave Decompositions, D.C. Programming, EM
- Training of deep architectures and large hidden variable models
- Approximation Algorithms
- Algorithms and Techniques (application oriented)
- Global and Lipschitz optimization
- Algorithms for nonsmooth optimization
- Linear and higher-order relaxations
- Polyhedral combinatorics applications to ML problems
- Combinatorial Optimization
- Optimization in Graphical Models
- Structure learning
- MAP estimation in continuous and discrete random fields
- Clustering and graph-partitioning
- Semi-supervised and multiple-instance learning
- Practical techniques
- Optimization software and toolboxes
- GPU, Multicore, Distributed implementations
- Applications close to machine learning
- Sparse learning, compressed sensing, signal processing
- Computational Statistics
- Large scale scientific computing
- Advanced optimization techniques
- core set based approximation schemes
- hashing based optimization
- optimization in statistics
- optimization on manifolds, metric spaces
- problems on cones
- polynomials, sums-of-squares, moment problems
Important Dates
- Deadline for submission of papers: 14h Oct 2013
- Notification of acceptance: 31st Oct 2013