Site menu:
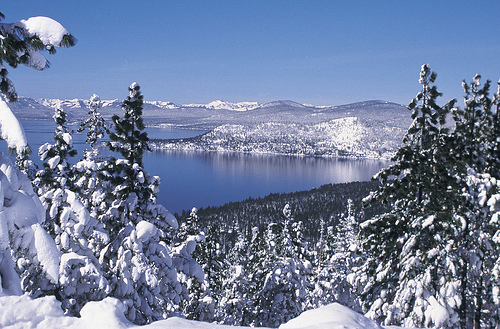
Optimization@NIPS
We welcome you to participate in the 5th NIPS Workshop on Optimization for Machine Learning, to be held at:
Lake Tahoe, Nevada, USA, Dec 8th, 2012
Accepted Papers
- Shai Bagon and Meirav Galun. A Multiscale Framework for Challenging Discrete Optimization
- Ohad Shamir. On the Complexity of Bandit and Derivative-Free Stochastic Convex Optimization
- Shai Shalev-Shwartz and Tong Zhang. Stochastic Dual Coordinate Ascent Methods for Regularized Loss Minimization
- Hua Ouyang, Niao He and Alexander Gray. Stochastic ADMM for Nonsmooth Optimization
- Martin Jaggi, Simon Lacoste-Julien, Mark Schmidt and Patrick Pletscher. Block-Coordinate Frank-Wolfe for Structural SVMs
- Le Hoai Minh, Ta Minh Thuy, Le Thi Hoai An and Pham Dinh Tao. DC Programming and DCA for clustering using weighted dissimilarity measures
- En-Hsu Yen, Nanyun Peng, Po-Wei Wang and Shou-De Lin. On Convergence Rate of Concave-Convex Procedure
- Kohei Ogawa, Ichiro Takeuchi and Masashi Sugiyama. A homotopy approach for nonconvex disjunctive programs in machine learning
- Jonathan Drake and Greg Hamerly. Accelerated k-means with adaptive distance bounds
- Victor Parque, Masakazu Kobayashi and Masatake Higashi. Explorit for Global Optimization
- Mehrdad Mahdavi, Tianbao Yang and Rong Jin. Online Stochastic Optimization with Multiple Objectives
- Silvia Villa, Saverio Salzo, Luca Baldassarre and Alessandro Verri. Convergence rates of nested accelerated inexact proximal methods
- John Moeller, Parasaran Raman, Avishek Saha and Suresh Venkatasubramanian. Fast Multiple Kernel Learning With Multiplicative Weight Updates
- Jason Lee, Yuekai Sun and Michael Saunders. Analysis of Inexact Proximal Newton-type Methods
- Huan Xu, Constantine Caramanis and Shie Mannor. Statistical Optimization in High Dimensions
- Huahua Wang and Arindam Banerjee. Alternating Direction Method with Bregman Divergences
- Prateek Jain, Praneeth Netrapalli and Sujay Sanghavi. Provable Matrix Sensing using Alternating Minimization
- Zaid Harchaoui, Anatoli Juditsky and Arkadi Nemirovski. Conditional gradient algorithms for machine learning
- Tom Schaul, Sixin Zhang and Yann Lecun. No more pesky learning rates
- Stephen Boyd, Corinna Cortes, Chong Jiang, Mehryar Mohri, Ana Radovanovic and Joelle Skaf. Large-scale Distributed Optimization for Improving Accuracy at the Top